The next time you meet someone who says they work with AI, ask them to describe their model.
Once there was the basic regression model, which plotted how an independent variable could affect a dependent one, a target variable. Fitting a line to a series of data points is called regression.
If the data is pretty evenly distributed, you will see a regression to the mean, a tending towards the middle.
Aim for the Middle
Averages can be deceptive if the data is not symmetrically distributed, but rather is skewed in one direction. Using the mean, we can say that practically everyone has a greater-than-average number of legs, which is 1.9999.
The barriers between the different professions that use modeling are breaking down. Today, we have much more compute and a lot more data to play with, so different groups of researchers have been developing strategies to create their own far more sophisticated models.
"The Art of Statistics: How to Learn From Data.”
David Spiegelhalter
Each discipline brings their own expertise and it is leading to a more "ecumenemical" approach to modeling, according to David Spiegelhalter’s "The Art of Statistics: How to Learn From Data.”
Four Types of Models
Statisticians still prefer rather simple mathematical representations for defining associations. Linear regression analysis fits the bill here.
Applied mathematicians create complex deterministic models, based on the scientific understanding of a physical process, intended to intended to “represent underlying mechanisms.” Weather forecasting is an example here.
Machine learning researchers tend to create complex algorithms used to make a decision or prediction, based on past examples. Book recommendations, works by this means. These are often black box models, that don’t offer an easy way to show how decisions were made. “Their internal structure is somewhat inscrutable,” Spiegelhalter writes.
Economists favor regression models that claim to reach causal conclusions.A model is more like a map than the territory itself. “All models are wrong, some are useful,” statistician George Box once observed.
Still they have their limitations. "Just because we act, and something changes, it doesn't mean we were responsible for the result. Humans seem to find this simple truth difficult," Spiegelhalter writes.
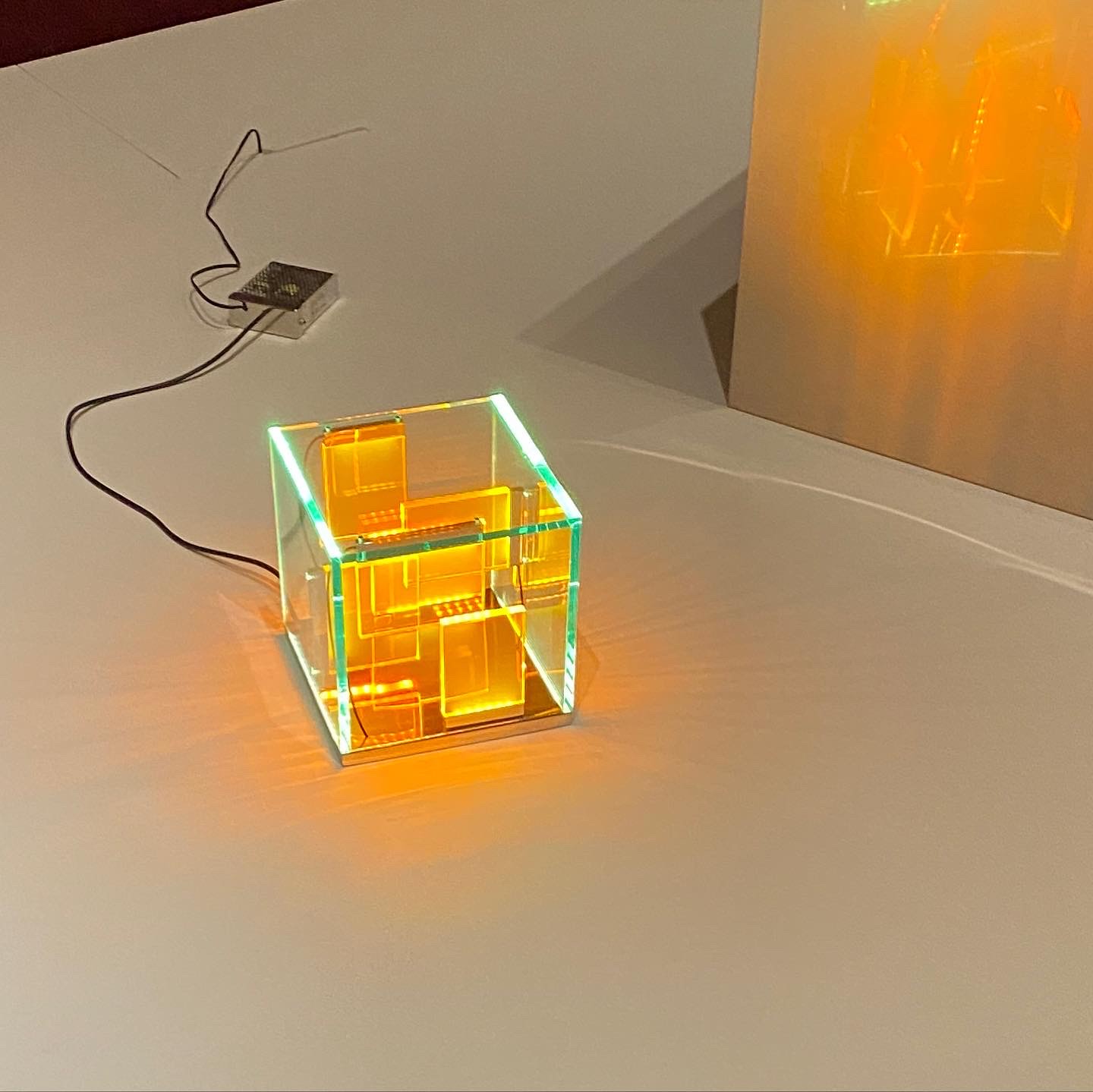